Keynote Speaker
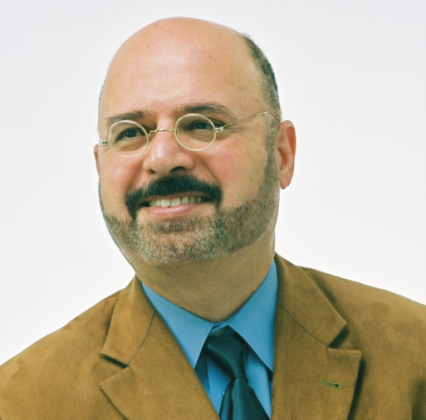
Dr. Hamido FUJITA, Professor (Life Senior Member IEEE)
Executive Chairman of i-SOMET Incorporated Association, JapanDistinguished Professor: Iwate Prefectural University, Japan
Professor at Malaysia-Japan International Institute of Technology, Universiti Teknologi Malaysia
Editor-in-Chief: Applied Intelligence (Springer)
Editor-in-Chief of International Journal of Healthcare Management (Taylor & Francis)
Emeritus Editor-in-Chief: Knowledge-Based Systems
Vice President of International Society of Applied Intelligence
Doctor Honoris Causa (Óbuda University, Hungary)
Doctor Honoris Causa (Timisoara Technical University, Romania)
Speech Title: Machine Learning Perspectives
Abstract: Training in Machine Learning is a crucial aspect that affects the credibility of the system in terms of performance and is employed for robust applications such as in healthcare systems. Machines or algorithms, in wide challengeable applications in security or vision or health care early predictions, learn from data. Nevertheless, in most cases, the extensive and unbalanced data and noise make it unreliable in prediction accuracy. Supervised machine learning is and was one of the aspects of providing artificial intelligence-based solutions. However, this is and was limited due to the difficulty of labeling big data and many crucial problems in weak relations and noise in data. Semi-supervised learning, for example, Multiview learning, could assist in solving these problems. In many published research, there are still problems in providing machine learning models that are unbiased and efficient in terms of robustness and resilience in data-driven systems. Multiclass classification still has problems in terms of clear definition in class classification, bias, imbalance and weak relations, making machine learning for multiclass classification insecure for classification or regression analytics. This causes limitations in applying such technology in medical applications and diagnosis prediction. In this lecture, I will outline these problems in our one-class classification project. These are related to providing more robust accuracy prediction with some uncertainty that can help us have more accurate classification and prediction. We have applied such findings in health care for heart sickness and seizure early prediction like in https://doi.org/10.1016/j.cmpb.2022.107277 and https://doi.org/10.1016/j.inffus.2023.102023
We also have deep learning models, which also have challenges related to evidential deep learning and fairness relative to data. There are important issues in expanding research in evidential deep learning, in which uncertainty prediction of variational Auto encoders can provide decisions on evidential distribution, which in turn helps to provide a measure of uncertainty in decision.
We currently have a research project titled “Healthcare Risk Prediction on Data Streams Employing Cross Ensemble Deep Learning”, which is supported by Japan Science Promotion Society (JSPS). In this project, we have employed one-class classification deep neural network for health care prediction. In this lecture I will outline of these perspective and discuss challenging trends.
Biography: Dr. Hamido FUJITA is Distinguished Professor of Iwate Prefectural University, Japan. He is also contracted Professor at Malaysia-Japan International Institute of Technology(MJIIT), Universiti Teknologi Malaysia. He is also Research Professor at University of Granada (Spain), Universiti Teknologi Malaysia, and HUTECH University Vietnam; Expert Excellence Professor at Shanghai University of Medicine & Health Sciences. He is currently the Executive Chairman of i-SOMET Incorporated Association, Japan. He is Highly Cited Researcher in Cross-Field for the year 2019 and 2020, 2021, 2022 in Computer Science field, respectively from Clarivate Analytics. He is Editor-in-Chief of Applied Intelligence (Springer), Editor-in-Chief of Healthcare Management (Tayler&Francis), and Editor-in-Chief of Knowledge-Based Systems (2010-2020) and Emeritus Editor of Knowledge-Based Systems. More details, please refer to his personal homepages via https://www.webofscience.com/wos/author/record/D-6249-2012 and https://scholar.google.com/citations?hl=en&user=MxzV1nQAAAAJ